Kenneth Hall
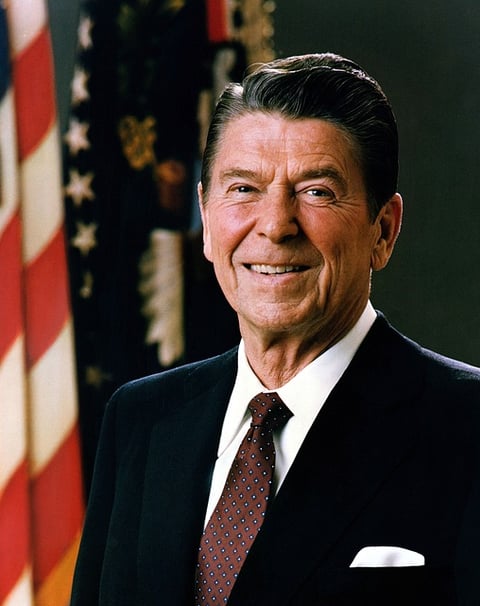
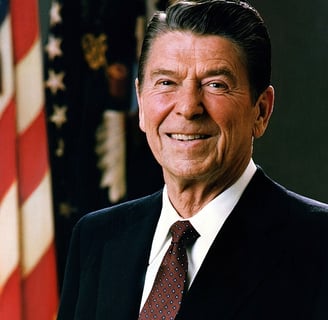
Name: Kenneth Hall
Role: AI-Driven Quality Inspection Specialist in Smart Supply Chain
Expertise: Leveraging AI Vision Technology for Product Defect Detection
Professional Summary:
Kenneth Hall is a highly skilled professional in the field of AI-driven quality inspection within smart supply chains, specializing in the application of AI vision technology for product defect detection. With a strong foundation in computer vision, machine learning, and industrial quality control, Kenneth is dedicated to transforming traditional inspection processes by integrating cutting-edge AI technologies. His work enhances product quality, reduces manufacturing defects, and ensures compliance with industry standards, ultimately improving operational efficiency and customer satisfaction.
Key Competencies:
AI Vision Technology for Defect Detection:
Develops advanced AI algorithms to analyze visual data, identifying defects such as cracks, discolorations, and misalignments with high precision.
Utilizes deep learning models to improve the accuracy and speed of defect detection across various product types.
Quality Inspection Automation:
Designs and implements AI-powered inspection systems to automate quality control processes, reducing human error and increasing throughput.
Integrates AI vision technology with production lines for real-time defect detection and immediate corrective actions.
Data Integration & Analytics:
Proficient in integrating and analyzing data from multiple sources, including high-resolution cameras, sensors, and production logs, to provide actionable insights.
Builds scalable data pipelines to process and interpret large datasets for continuous improvement of inspection systems.
Machine Learning Expertise:
Builds and optimizes machine learning models for quality inspection applications, including image classification, object detection, and anomaly detection.
Stays updated with the latest advancements in AI and computer vision to drive innovation in quality control.
Cross-Functional Collaboration:
Collaborates with quality assurance teams, production managers, and business leaders to align AI solutions with organizational goals.
Provides training and support to ensure the successful adoption of AI tools.
Career Highlights:
Developed an AI-powered inspection system that reduced defect rates by 25% for a leading electronics manufacturer.
Designed a real-time defect detection framework that improved inspection speed by 30% for a global automotive supplier.
Published influential research on AI applications in quality inspection, earning recognition at international manufacturing conferences.
Personal Statement:
"I am passionate about leveraging AI vision technology to revolutionize quality inspection, ensuring that products meet the highest standards of quality and reliability. My mission is to create data-driven solutions that enhance manufacturing processes, reduce defects, and deliver superior products to customers."
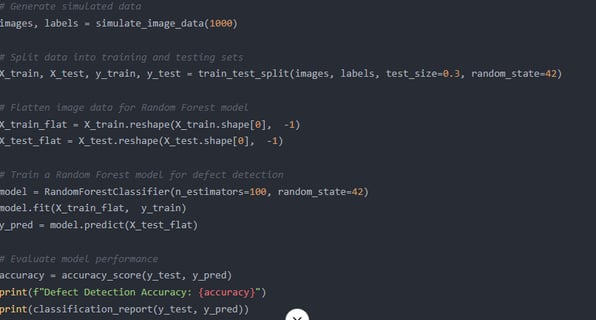
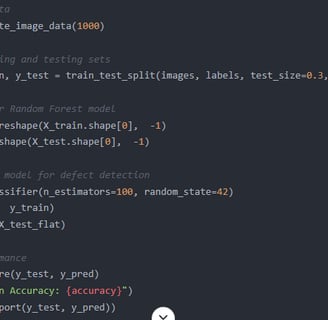
Fine-Tuning Necessity
Fine-tuning GPT-4 is essential for this research because publicly available GPT-3.5 lacks the specialized capabilities required for analyzing complex visual data and detecting product defects. Visual inspection involves highly domain-specific knowledge, intricate defect patterns, and nuanced classification criteria that general-purpose models like GPT-3.5 cannot adequately address. Fine-tuning GPT-4 allows the model to learn from visual inspection datasets, adapt to the unique challenges of the domain, and provide more accurate and actionable insights. This level of customization is critical for advancing AI’s role in quality control and ensuring its practical utility in real-world manufacturing scenarios.
Past Research
To better understand the context of this submission, I recommend reviewing my previous work on the application of AI in visual inspection, particularly the study titled "Enhancing Product Defect Detection Using Machine Learning Models." This research explored the use of convolutional neural networks (CNNs) and transfer learning for improving defect detection accuracy. Additionally, my paper "Adapting Large Language Models for Domain-Specific Applications in Quality Control" provides insights into the fine-tuning process and its potential to enhance model performance in specialized fields.